ASIMoW
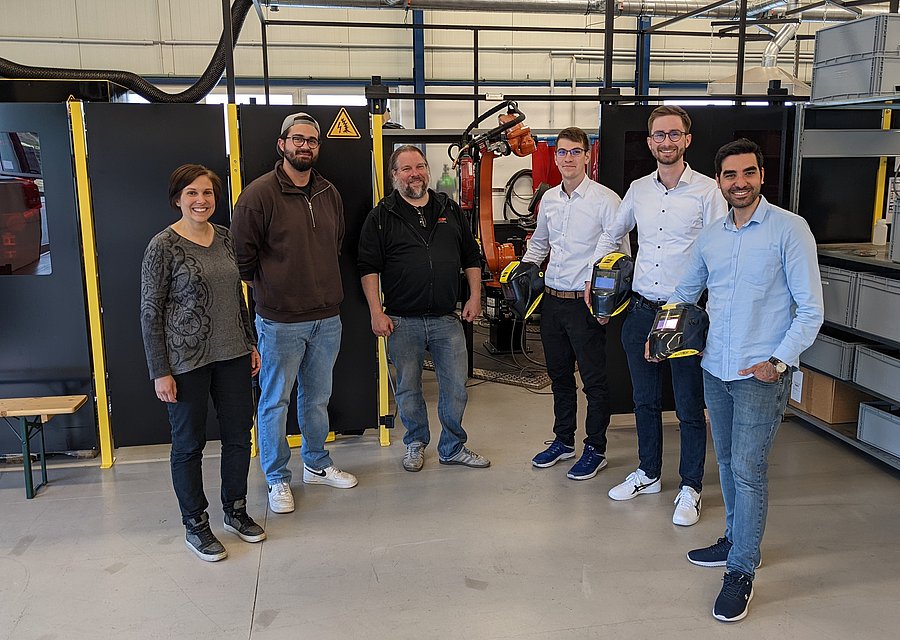
The team behind the ASIMoW project (from left to right): Marion Purrio, David Dentzer and Guido Buchholz (FEF GmbH), Yannik Hahn, Robert Maack and Hasan Tercan (Chair of TMDT).
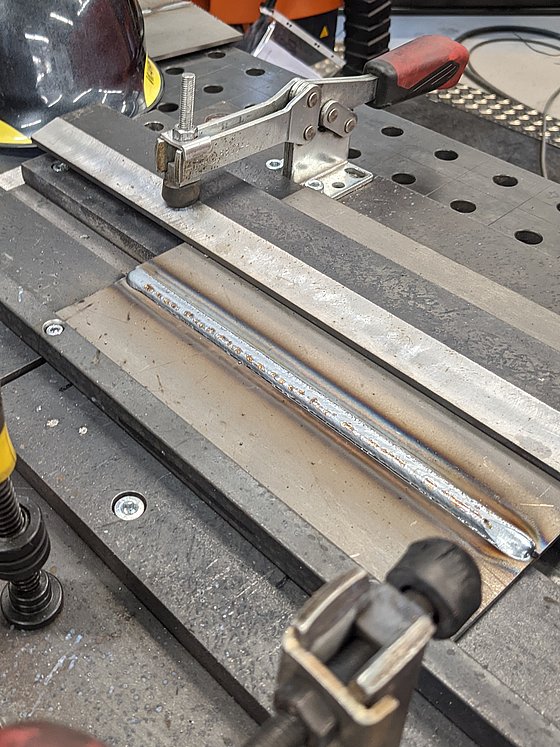
Lawed weld seam in gas metal arc welding
Analysis system for quality-motivated inline monitoring for welding processes based on artificial intelligence methods
The group of joining technologies represents a key position in the context of a modern manufacturing environment. The quality of structural joints in particular has a lasting impact on the operational reliability and availability of complex products. Joining technologies are characterized by a high degree of inherent complexity, which can usually only be addressed by empirical methods. In particular, fusion welding processes involving several phases of joining partners and whose thermo-physical conditions have a lasting influence on the current process situation require expertise and experience.
The challenge of robust joint quality estimation is made all the more complex by the diverse variations of welding equipment used in the landscape of industrial welding processes. The one process configuration that produces good joined joints in a particular application environment cannot be readily transferred to other processes. Both the reconfiguration of the welding equipment and the accumulation of additional expert knowledge represent a considerable expenditure of time and lead to significant additional costs due to additional test runs.
Against this background, the objective of the research project "ASIMoW" is the development, testing and implementation of Deep Learning methods for inline quality prediction in welding processes as well as the research and development of Continuous Learning methods in order to be able to use the trained models sustainably and efficiently across process variants. The models will be able to evaluate the current process state based on transient and heterogeneous data from the welding process - including manipulated variables and sensor data - and derive a quantitative prediction of the expected weld quality. Using continuous learning, it will be possible to adapt models that have already been trained and used in response to changing process conditions and to train them continuously on a small amount of process data. The developed methods will finally be combined and evaluated in a demonstrator. Using the demonstrator, welding processes with different process configurations and joint types can be carried out and evaluated under real conditions.
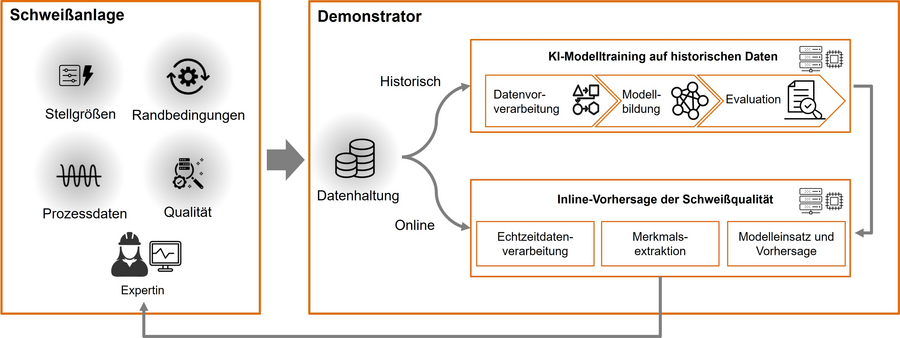
Target architecture for AI-based online quality prediction in welding