Machine Learning-based Predictive Quality in Manufacturing Processes
Hasan Tercan's dissertation investigates quality predictions in manufacturing processes using machine learning. He introduces a process model called MERLIN, which is complemented by methods such as transfer learning and continuous training to increase the data efficiency of the learning models.
We interviewed Mr. Tercan about his thesis.
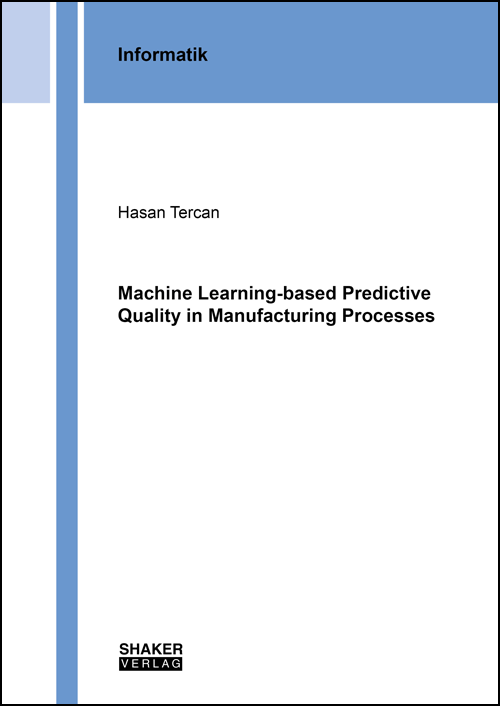
What was the context of your dissertation? What projects or other factors particularly influenced your dissertation?
The dissertation emerged from research findings that I had accumulated over several years in various research projects. An important project that shaped my work was the Cluster of Excellence "Integrative Production Technology for High-Wage Countries", in which I participated during my time at RWTH Aachen. This initiative laid the foundation for my research on predictive quality. In addition, my involvement in this cluster allowed me to delve deeply into Simulation-to-Reality Transfer Learning, which allows us to use simulation data to train ML models. In addition, my engagement in R&D projects with manufacturing companies provided me with valuable insights and opportunities to validate my work through real-world applications in these projects.
How does your work contribute to the field of research?
The work contributes significantly to the successful implementation of predictive quality in real manufacturing processes. In manufacturing facilities, collecting training data for machine learning applications remains a significant challenge. In addition, understanding how machine learning models perform under real, changing process conditions is critical. The dissertation addresses these challenges by developing transfer learning and continual learning approaches. Such approaches are relatively unexplored in the field of predictive quality.
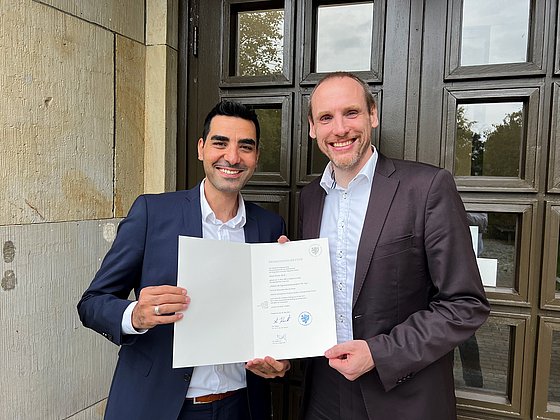
Certificate handover with Prof. Meisen
What does the future hold for you and the topic?
The topic of predictive quality remains central to the TMDT and is being explored in various projects and applications, such as real-time quality prediction in metal arc welding and spatially resolved quality estimation in plastics welding. As head of the Industrial Deep Learning research group, I look forward to pursuing these and other topics at TMDT.