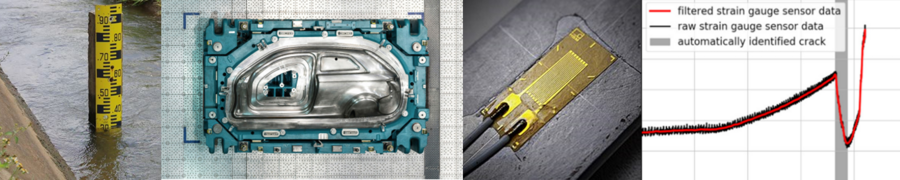
Analysis of time series data from sensor systems.
Interpretable Learning Models
Our research group Interpretable Learning Models (ILM) focuses on data driven approaches and corresponding analysis methods for time series and image data in production environments. Our goal is to facilitate the application of state-of-the-art learning models in complex and challenging scenarios such as condition monitoring, predictive quality, or predictive maintenance. Our main research topics cover the facilitation of transparency and interpretability of trained learning models, with a strong focus on artificial neural networks and deep learning models, to soften their inherent black-box character enabling their robust and reliable application in these environments. To this end, we rely on a broad variety of classical analytical methods from the field of signal processing, and modern learning mechanisms from the current state of the art in deep learning research. Additionally, we take inspiration from the research field of neuroscience aiming to promote a new perspective on artificial learning models as objects of interest in large scale empirical studies. We strongly believe that interactive and visual exploration of learning models and their corresponding data is the key for better transparency and interpretability. We envision the future of artificial learning models to be just as tangible, accessible, and easy to investigate as common everyday objects in the palm of our hands.
Areas of Research
Prediction of temporal progressions of sensor data, for example for the use case predictive maintenance.
Pattern recognition in temporal progressions of sensor data, for example for the use case predictive quality.
Extraction of typical patterns in temporal progressions of sensor data and detection of irregular progressions and anomalies.
Reconstructing chronological histories of sensor data from underlying and correlating sensors for replacing hardware sensors with AI-based software sensors
Areas of Application
Typically from temperature, pressure, speed, voltage, current, power, or strain gauge sensors.
Permanent monitoring and condition determination of machines and production lines by means of sensor systems.
Predictive quality assessment of components and products through the predictive analysis of sensor data.
Predictive planning of machine maintenance by determining the aging process of wear parts through predictive analysis of sensor data.