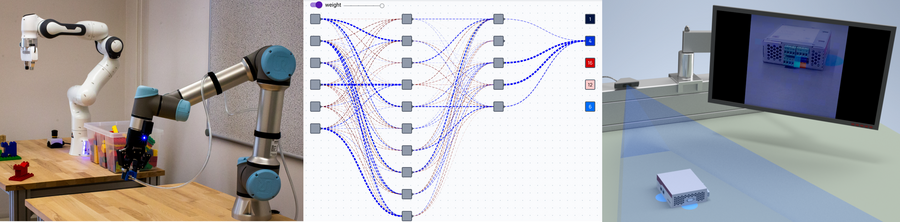
Deep learning for industrial applications
Our research focuses on the development and testing of Deep Learning methods for use in industrial processes and services. On the one hand, our research aims at establishing Deep Learning as an enabler of new services such as Predictive Quality and Predictive Maintenance. On the other hand, we investigate reinforcement learning methods for training intelligent, self-learning agents to solve planning tasks. Central objects of investigation are the formalization of real use cases as learning problems and their solution under consideration of industrial evaluation criteria such as reliability, robustness and accuracy.
Furthermore, we address the question of how data-efficient and sustainable deep learning models can be developed for use in the manufacturing industry. Our focus here includes, in particular, bridging the reality gap of simulations using transfer learning methods as well as the continuous training of deep learning models across process and system changes.
Areas of Research
Suitability of reinforcement learning methods (including Q-learning, soft actor-critic, PPO) for real-world planning and control problems.
Design and implementation of data-based predictive learning models (e.g., artificial neural networks) for real-world prediction problems.
Development of deep learning methods for fault and anomaly detection in industrial processes.
Exploring and testing transfer learning and continual learning paradigms to generate data-efficient and robust learning models.
Areas of Application
Intelligent and adaptive planning of production processes in compliance with real evaluation variables (e.g. adherence to schedules) and boundary conditions.
Intelligent and collision-free trajectory planning for controlling industrial robots in real assembly and joining scenarios.
Data-driven quality prediction in production and manufacturing processes based on machine learning and deep learning methods.
Data-driven prediction of operating conditions in industrial plants and processes based on machine learning and deep learning methods.