Prof. Dr.-Ing. Tobias Meisen
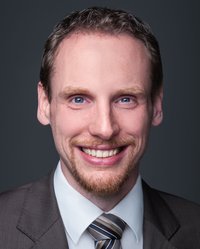
Professor of Technologies and Management of Digital Transformation
Area of Research:
- Deep and Machine Learning
- Deep Reinforcement Learning
- Explainable and Transparent Artificial Intelligence
- Knowledge Graphs
- Semantic Interoperability
Biography
Univ.-Prof. Dr.-Ing. Tobias Meisen has been Professor of Technologies and Management of Digital Transformation at the University of Wuppertal since September 2018. He studied computer science with a focus on data mining and data management and received his doctorate (Dr.-Ing.) with distinction. From 2015 to 2018, he held a junior professorship at RWTH Aachen University, where he conducted research within the DFG Cluster of Excellence “Integrative Production Technology for High-Wage Countries” and served as managing director of the Institute of Information Management in Mechanical Engineering.
His research focuses on digital transformation and modern information management in an increasingly connected world. A particular emphasis lies on the development of data-driven systems based on machine learning and deep learning methods, specifically tailored for industrial applications. In contrast to many traditional AI approaches that rely on large, centralized datasets, his work addresses the challenges of real-world industrial environments, where data is often distributed, heterogeneous, or incomplete. The goal is to enable robust, adaptive systems that can automate processes, support decision-making, and enhance human-machine interaction under such conditions. In addition, he investigates the structured acquisition, integration, and management of data through the development of knowledge graphs, which serve as a foundation for transparent and trustworthy industrial AI systems.
Tobias Meisen is the spokesperson of the Interdisciplinary Center for Machine Learning and Data Analytics (IZMD) at the University of Wuppertal and chair of the board of the affiliated institute SIKoM, dedicated to systems research in information, communication, and media technologies. He is also a member of the scientific advisory board of the Center for Advanced Internet Studies (CAIS). Since February 2025, he has been contributing his academic expertise as an expert member of the parliamentary commission of inquiry “Artificial Intelligence – Towards a Smart State in a Digital Society” of the State Parliament of North Rhine-Westphalia.
As co-founder of HotSprings GmbH, which was later integrated into umlaut and is now part of Accenture, Tobias Meisen brings extensive experience at the intersection of academic research, technological innovation, and practical application. His work has received several recognitions, including best paper awards and the Young Researcher Award as part of Germany’s Excellence Initiative. He is the author and co-author of numerous scientific publications and is actively engaged in national and international research and development projects in collaboration with academic and industry partners.
Publications
- 2024
- Hahn, Y., Maack, R., Buchholz, G., Purrio, M., Angerhausen, M., Tercan, H., & Meisen, T. (2024). "Quality Prediction in Arc Welding: Leveraging Transformer Models and Discrete Representations from Vector Quantised—VAE" in Proceedings of the 33st ACM International Conference on Information & Knowledge Management .
ISBN: 979-8-4007-0436-9
- Jantunen, M., Meyes, R., Kurchyna, V., Meisen, T., Abrahamsson, P., & Mohanani, R. (2024). "Researchers' Concerns on Artificial Intelligence Ethics: Results from a Scenario-Based Survey" in Proceedings of the 7th ACM/IEEE International Workshop on Software-intensive Business , New York, NY, USA : ACM 24—31.
ISBN: 9798400705717
- Weiss, M., & Meisen, T. (2024). "Reviewing Material-Sensitive Computed Tomography: From Handcrafted Algorithms to Modern Deep Learning" , NDT , 2 (3), 286—310.
- Weiss, M., Brierley, N., Schmid, M., & Meisen, T. (2024). "Simulation Study: Data-Driven Material Decomposition in Industrial X-ray Computed Tomography" , NDT , 2 (1), 1—15.
- Brune, M., Meisen, T., & Pomp, A. (2024). "Survey of Deep Learning-Based Methods for FMCW Radar Odometry and Ego-Localization" , Applied Sciences , 14 (6), 2267.